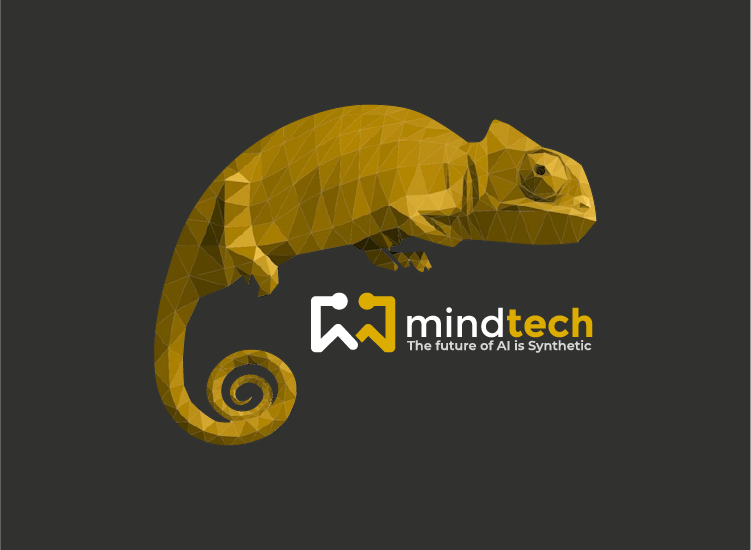
Share article:
Tags:
Every ML training project runs into the data wall sooner rather than later. The truism that ‘he who has the most data wins’ applies with equal force whether you are a resource-rich giant or a hungry startup and as a consequence, the average project spends more than 80% of its time on acquiring, labelling and curating data. Even for big companies with plenty of cash, this presents a huge obstacle to progress so it is no surprise that project managers are turning to synthetic data to fill the need. This doesn’t, however, necessarily make the problems all go magically away. Using a simulator will solve many of the most pressing problems but unless careful attention is given to the whole workflow, an entirely new set of problems can quickly grow out of control.
With the right tools, however, we can stop looking at data creation as a problem and start looking at it as an opportunity.
With classical real-world data collection and labelling, the expenses in both time and cost are back-loaded. Although a recording session can be planned and completed in a few weeks, labelling the resulting data can take many, many man-months. With synthetic data, that part of the workflow comes more or less for free but at the cost of moving the heavy lifting to the earlier parts of the process and this is where the right tool makes all the difference.
The task of building a synthetic world starts with the asset creation and moves through world building and scenario programming before a single simulation run can begin. In the games world, this is where the vast majority of the costs lie so it is no accident that early efforts at acquiring synthetic training data centered around leveraging the expensively produced assets and gameplay of Grand Theft Auto: the teams doing that research had neither the skills nor the budget to create anything remotely comparable.
This free ride was quickly and emphatically closed down by the owner of GTA, which gives an indication of the value placed on assets by the game industry. It should also give the data science community a hint as to where they can create value for themselves.
For a data science team, being able to leverage the wide ecosystem of assets created for general visualization purposes is key to reducing the overhead of asset acquisition. This is the reason the Chameleon platform workflow starts with import of standard FBX assets into a coherent environment but the importance of starting the workflow at this point goes well beyond cost reduction and has implications for the scope and value of the entire data creation pipeline.
In a synthetic data creation environment, it is important to remember that the raw assets not the finished images are the source data and that the process of provisioning and curation, including labelling, starts at the point of import. This is a significant change from the existing mindset which regards acquired data as a fixed quantity that can be augmented in some very limited ways to one where the source data now becomes malleable and can be tuned and modified to suit the application during the creation process.
This simple concept of data malleability puts an incredibly powerful tool into the hands of the data science community and opens the door to a completely new way of working where training data now becomes another variable that can be tweaked during the training process.
The Chameleon platform recognizes the primary importance of this source data by providing an asset management tool that takes care of the import, provisioning, labelling and storage of these raw assets. Once imported into the system, these assets become discoverable and trackable using the same user-defined categorization and tagging scheme that will later on be employed by the training framework. As a result, not only will the source data for any particular simulation run be easily retrieved for data provenance purposes but curation/creation of new data can be made an automatic process driven by the results of previous training passes.
One of the more interesting and significant trends over the last couple of years has been the ongoing ‘whiteboxing’ of networks where tools now exist to look inside a network and examine, layer by layer, just what factors are influencing network performance. These tools are most often used to guide the tweaking of hyperparameters but they can equally well be used to judge whether more data is needed and give guidance on what it needs to be.
This combination of data malleability with network transparency opens up the prospect of iterative network training that includes the source data as one of the variables and from this powerful new ways of optimizing networks will emerge.
—
Editors Note: This is the first in a series of posts by MIndtech’s VP Engineering, Peter McGuinness