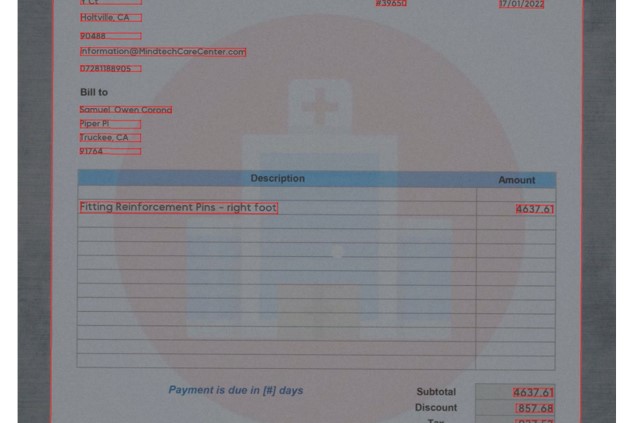
Share article:
Tags:
Optical Character Recognition (OCR) has been around for decades, allowing users to for example, convert printed into machine-readable text. However, as technology has advanced, the limitations of traditional OCR methods have become increasingly apparent. This has led some to question whether OCR is still a viable technology. However, recent developments in synthetic data offer a promising solution to these limitations.
Limitations
Traditional OCR methods require a large amount of labelled training data to accurately recognise text. This can be time-consuming and costly, particularly when dealing with handwritten text, which can be more difficult to recognise. Additionally, OCR is often used to extract data from historical documents, which may be faded or damaged. Traditional OCR methods may struggle to accurately recognise text in such cases.
Where can synthetic data be useful?
However, there are several areas where synthetic data can be very useful in OCR and related fields:
Scanning of old documents: When old documents are scanned, they are likely to be aged and potentially damaged. Synthetic data can generate images of these documents, accounting for variation in a number of visual factors, in turn allowing OCR models to be trained on more representative data.
Mobile scanning: Scanning is no longer always performed with controlled flatbed scanners but often with mobile phone cameras, which can result in a blur due to depth-of-field differences, camera shake, documents being non-flat, obstructions in the way such as fingers covering the documents, and background items on desks. Many documents may have been folded or crumpled, such as receipts stuffed into wallets and then removed for automated expense processing.
Moving from OCR to deep document understanding: With the advancement of machine learning, we can move from OCR to deep document understanding. When a document is scanned, we want to automatically identify addresses, dates, total costs, and more.
The promise of synthetic data
By using synthetic data, OCR models can be trained more efficiently and accurately and can be tailored to specific use cases. Mindtech’s synthetic data platform, for example, offers several advantages in this regard:
3D engine: Mindtech’s platform includes a full 3D engine, allowing it to create appropriately creased and damaged documents.Camera variation: Mindtech’s platform can vary the camera position and model depth-of-field blur, camera shake, off-axis photos, and more.Occlusions and background detritus: Mindtech’s platform can model occlusions and background detritus that are present in real-world scans.Tabular and visual data: Mindtech’s platform can combine synthetic tabular and visual data, allowing it to create massive numbers of documents without issues of Personally Identifiable Information (PII).
As technology continues to advance, it’s likely that we will see more applications of synthetic data in OCR and other fields. The limitations of traditional OCR methods have led some to question whether OCR is still a viable technology. However, recent developments in synthetic data offer a promising solution to these limitations. By using synthetic data, OCR models can be trained more efficiently and accurately and can be tailored to specific use cases.
Is OCR Dead? Exploring the Potential of Synthetic Data was originally published in MindtechGlobal on Medium, where people are continuing the conversation by highlighting and responding to this story.